The adult human brain contains ∼86 billion neurons (1). Zooming into its cellular and subcellular details to reveal different aspects of neuronal connectivity is a key area of research. However, to link the different spatial scales from the synaptic level (at nanometer range) through single neurons and glial cells (at the micrometer level) to the whole organ is most challenging. Recently, the connectome of Caenorhabditis elegans, with its 302 neurons, has been characterized, and a complete structural-functional model has been proposed (2). A comparable level of detail of the human brain connectome is still a long way off. As such, decoding the human connectome, the mechanisms of signal transduction, and relationships to brain function are linked to exponentially growing challenges in advanced computational and storage technologies, which in turn may lead to creative solutions beyond neuroscience.Challenges of scanning time, storage technology, and data processing do not start with studies of the human brain but are important considerations when studying brains of vertebrates and even invertebrates (see the figure). For example, the reconstruction of the synapse connectivity of an adult fruit fly brain, with ∼100,000 neurons, culminated in ∼21 million camera images and a dataset of 106 terabytes (3). Recently, a fragment of the human cerebral cortex, a volume of ∼1 mm3, was reconstructed in three dimensions, corresponding to a data volume of ∼1.4 petabytes (4). Although 1 mm3 is a tiny portion of the cerebral cortex and represents ∼0.00007% of the total brain volume, a high-speed multibeam electron microscope was required to collect these data with a scan time of 326 days. Such research is conducted with the motivation that insights into the detailed architecture of the brain may reveal a new understanding of cortical networks and provide new quantitative data that describe tissue properties, with implications for brain activity.This type of analysis of human brain tissue is an important complement to studies of brains from other species because there are considerable differences between them, particularly connectivity. For example, the volume of white matter of the cerebral cortex, which contains axons that support long-range connectivity, increases faster during mammalian evolution than gray matter (which contains neuronal cell bodies) (5). The ratio of long to short connections has consequences for electrophysiological properties and, thus, for brain function (6). However, studying the full extent of axons and their synapses, which may be several centimeters from their cell bodies, represents a much more substantial problem in human brains than in those of rodents or invertebrates.Magnetic resonance imaging (MRI) (e.g., diffusion MRI) is capable of addressing long-range connections and revealing connectivity patterns, including functional connectivity in the living human brain, but image voxels are in the millimeter range. This resolution is far below the spatial resolution necessary to detect single axons and therefore is prone to lead to ambiguities inherent in tract reconstruction (7). Moreover, brain connectivity includes phenomena such as collateralization (one axon may target multiple areas), convergence, reciprocity, or spatially separate dendritic arbors, which are considered key in understanding connectivity (8) but are not accessible with MRI.Consequently, there is a discrepancy between macro- and microconnectome scales (9). Different approaches have been proposed to bridge the two worlds: For example, a combination of diffusion MRI and high-resolution optical imaging of fluorescently labeled neurofilaments in brain tissue cleared using the CLARITY technique has recently been explored in the same tissue block to allow more robust multimodal MRI-CLARITY comparisons (10). In another study, a tissue block from the human hippocampus was imaged with anatomical and diffusion MRI, three-dimensional polarized light imaging [3D-PLI; (11)], and two-photon fluorescence microscopy to bring together information of the fiber architecture of the hippocampus at different spatial scales and to represent it in a common reference space, the BigBrain model (www.ebrains.eu). These data show the perforant pathway, which does not represent a uniform tract but rather a multicomponent system that originates in the entorhinal cortex with many thin axon fiber bundles (in the range of 20 µm) and projects to the cornu ammonis and subiculum (12). 3D-PLI is a microscopic technique that allows decoding of the fine architecture of the perforant pathway, which has a central role in learning and memory, as well as in the pathogenesis of Alzheimer’s disease. Methodologically, the combination of different techniques helps to cross-validate findings. Practically, it paves the way to combine high-resolution region-of-interest analysis with whole-brain studies.Atlas methods can, in a systematic manner, serve as a bridge of macro- and microscales by integrating data from ultrahigh-resolution studies of single cells and their connections to small regions of interest into a common microscopical reference space. BigBrain represents such an anatomical model at 20-mm isotropic resolution, which is slightly higher than the resolution required to see details of cell morphology. It is based on 7404 histological, cell body–stained sections; the original dataset is ∼1 terabyte (13). BigBrain provides a template to integrate findings of cortical layers and even sublayers with data on whole-brain structure, connectivity, and function. Data integration in an atlas combines the advantages of a bottom-up approach that starts with cellular characteristics and connections to build up to the macroscopic scale with the advantages of a top-down perspective that starts with behavioral function and underlying large-scale networks to better understand the properties of components, that is, cells and their circuits. Both approaches are required to provide mutual information and to constrain predictions.It is a gigantic challenge to process and analyze entire human brains at cellular resolution. At present, it is not within reach to go down to individual axons at the whole-brain level. However, what would it mean computationally to track axons at the whole-brain level, assuming for simplicity that an axon connects two neurons? To compute the course of fibers at the whole-brain level in the millimeter range based on diffusion MRI of postmortem brains would mean to optimize 4 × 105 spin directions. This will require ∼130 megabytes of storage and 1 day of computation time on a desktop computer.The analysis of axons at the level of 60-µm isotropic resolution for a whole human brain using 3D-PLI (11) would require 8.3 terabytes of storage and several days on existing supercomputers to optimize 2 × 1010 spins. Such large datasets also create substantial challenges for the visualization of data. Paraview, for example, based on the open-source software VTK (www.paraview.org), can use parallel graphics processing units (GPUs) and has been applied to render and visualize 3D-PLI data. Going further and optimizing fiber orientations obtained with 3D-PLI at 1.3-µm inplane resolution (i.e., single axons) with 1013 spins would result in a storage demand of 3.2 petabytes and years of computation. This is not possible with current petascale technology but can be accomplished with future exascale computing power, that is, computers capable of executing 1018 floating point operations per second (i.e., 1 exaflop). Handling such large datasets, however, creates massive computational demands at the level of input-output. More efficient input-output procedures and algorithms are emerging, which should help, but the computational challenges are still extraordinarily high…
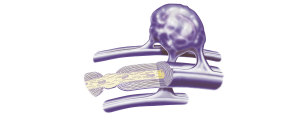